Prologue: The old woman and the five elephants.
Svetlana Geier is in her eighties. “I’m too old for breaks,” she says to her collaborator, turning down a cup of tea. There is work to be done. She has been joined at her home in Freiburg by Frau Hagen, a retired executive secretary who has brought a typewriter along, a vintage Olympia International. Sitting across the desk, Geier starts dictating. “From the notes of a young man…” It’s the title page of Dostoyevsky’s The Gambler, which Geier is translating from her native Russian into German.
The scene changes: Frau Hagen has been replaced by Herr Klodt, a musician. Geier sharpens two pencils and lines up her typewritten copy of the draft chapter. Herr Klodt is reading aloud from a second, identical copy. He listens for the sounds of the translation, and quizzes Geier about some of her word choices. It is a spirited and erudite conversation. She notes down his suggestions in pencil.
These snapshots are recorded in The Woman With the Five Elephants, a documentary that Vadim Jendreyko completed in 2009, the year before Svetlana Geier’s death. The five elephants are Dostoyevsky’s great novels (Crime and Punishment, The Idiot, The Brothers Karamazov, Demons and The Gambler), which Geier had been commissioned to translate in 1990, though she was already in her late sixties. And translate them she did, over the course of nearly twenty years, without so much as touching a computer along the way. Her work practice exemplifies a traditional view of the translator-as-artist: a patient, skilled practitioner whose job is nearly as difficult and creative as that of the original author.
Today, less than a decade after Geier’s death, I can download an electronic copy of any of Dostoyevsky’s novels, in Russian, and have it machine-translated into German, English or my first language, Italian, in a matter of seconds. But can a piece of software do the work of Svetlana Geier? One worked for two decades at her desk, calling upon a lifetime of professional expertise and knowledge of literature and the world. The other converted the Russian words of Dostoyevsky into digits and sent those digits to a system running on a battery of graphics processing units housed half a world away. That system has no built-in concept of culture or meaning, yet it almost instantaneously returned an equal number of strings of words in English, German, or Italian. And called it a “translation.”
Work is being done, but a machine is doing it; I have a translation, but where is the translator?
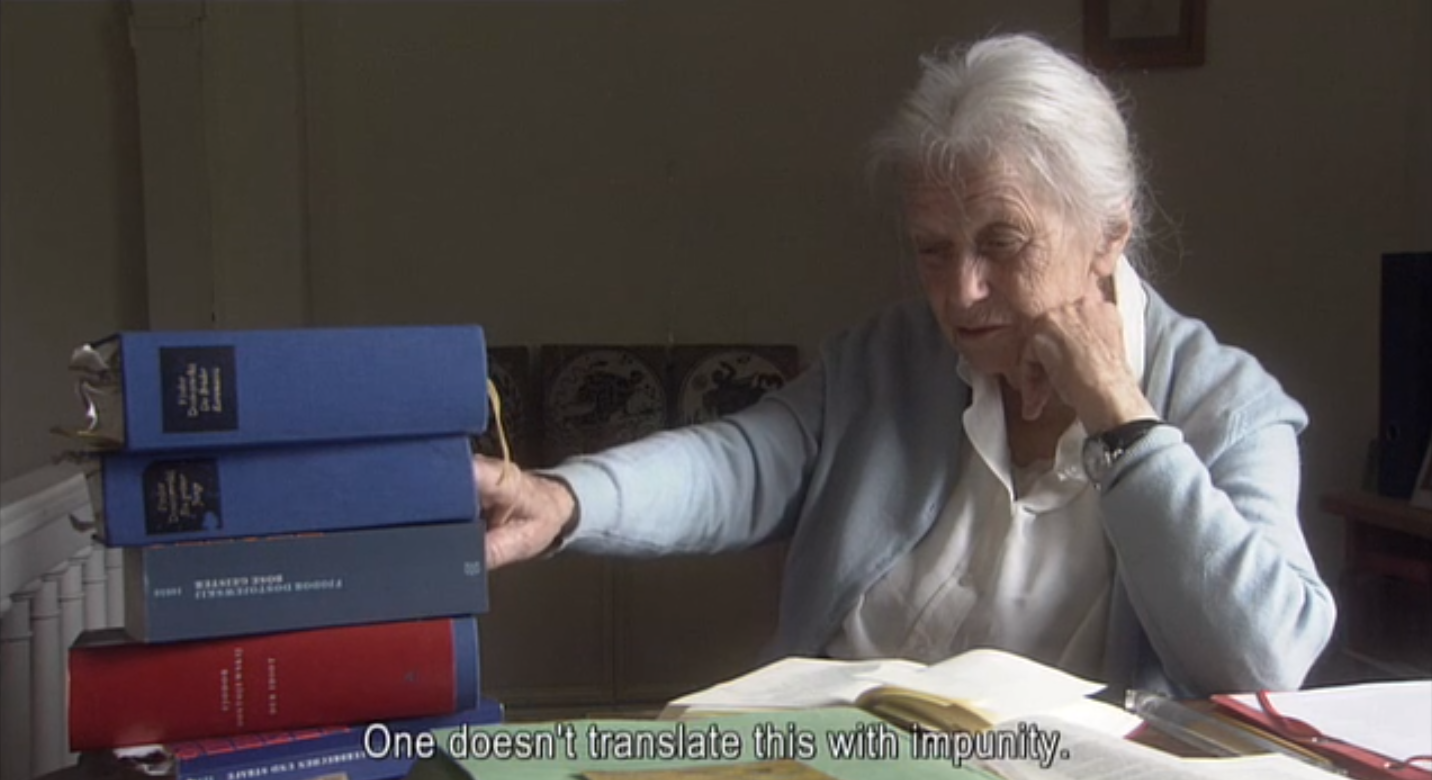
What words mean
This is a story about words that change into other words and about words that change meaning when they stay the same.
I call myself a “translator.” I have been a translator since 1990, the same year Svetlana Geier accepted her elephantine commission. But since that time, the words translation and translator have come to have less and less to do with humans. Machines are taking the central role: the words “translation” and “translator” are associated more and more with automated services such as Google Translate and Microsoft Translator. In turn, translation agencies have become language-service providers, while translators have become “linguists,” or “language specialists.” They are the experts that oversee a process, rather than the prime agents of that process.
There is nothing sinister about words changing their meaning, as any translator will tell you; didn’t the word “computer” once refer to a person who carried out calculations? The phrase “computing-machine” has disappeared, and perhaps the word “machine” will become redundant in the phrase “machine translation.” Perhaps carrying out a translation by hand (or by mouth) will seem as much a waste of time as carrying out a long calculation on paper.
Perhaps. But while we’re not there yet, the fact that such an outcome is even conceivable is enormously important. The act of translation isn’t very far from the act of invention: To translate is not very different than to speak, or to think. To propose that machines will soon be able to fulfill this function as competently as any human has profound implications on our shared social view of intellectual labor, and whether it should remain the preserve of actual people.
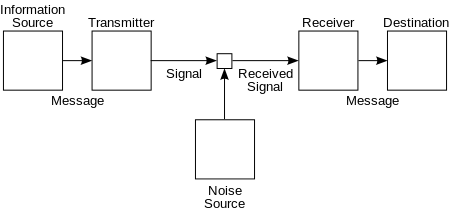
The history of machine translation until the day before yesterday
Warren Weaver thought of it first. In a letter to fellow mathematician Norbert Wiener, dated March 4, 1947, he observed that translation was “a most serious problem, for UNESCO and for the constructive and peaceful future of the planet” and began to speculate “if the problem of translation could conceivably be treated as a problem in cryptography. When I look at an article in Russian, I say ‘This is really written in English, but it has been coded in some strange symbols. I will now proceed to decode.’”
The reference to Russian was not completely casual: Weaver and Wiener had fought in the war as scientists, in cryptography and what would come to be called cybernetics. To think of translation as cryptography makes foreign languages something like enemy code. Two years later, Weaver fleshed out his initial suggestion with a concrete proposal:
If one examines the words in a book, one at a time through an opaque mask with a hole in it one word wide, then it is obviously impossible to determine, one at a time, the meaning of words. “Fast” may mean “rapid”; or it may mean “motionless”; and there is no way of telling which. But, if one lengthens the slit in the opaque mask, until one can see not only the central word in question but also say N words on either side, then, if N is large enough one can unambiguously decide the meaning.
One could quarrel on whether ambiguity can be removed from language, but even so, Weaver’s intuition very accurately described present-day machine translation. The phrase “if N is large enough” is the key: by leveraging incomprehensibly vast corpora of multilingual texts—the Rosetta Stones of our age—systems like Google Translate infer meaning from context. And as some claim to have “bridged the gap between human and machine translation” these claims have been amply echoed in the press: In an in-depth feature, for example, Gideon Lewis-Kraus argued that “human-quality machine translation is not only a short-term necessity but also a development very likely, in the long term, to prove transformational”: as the “first step toward a general computational facility with human language,” machine translation “would represent a major inflection point — perhaps the major inflection point — in the development of something that felt like true artificial intelligence.”
It is worth lingering on Lewis-Kraus’s piece, which is full of fascinating insights and detail; by placing the latest iteration of Google Translate at the center of the AI arms race between the largest tech companies in Silicon Valley, he lucidly diagnoses the real revolution in Silicon Valley: “institution-building—and the consolidation of power—on a scale and at a pace that are both probably unprecedented in human history.” But in the weeks he spent at the Googleplex, in the company of its designers, Lewis-Kraus perhaps gets swept up in their enthusiasm for what Translate might do, without reflecting on the history of claims similar to those made by its makers and marketers.
The proposition that the great breakthrough is “just around the corner” is a constant in the history of machine translation. The researchers who developed the first IBM system at Georgetown University predicted, in 1954, that translation would be solved “within the next few years.” Their machine adequately rendered 49 carefully-selected sentences from technical Russian into English. These early systems were rule-based: computers were equipped with a dictionary and taught rules on how to arrange and decline words correctly in the target language. Grammar and fluency were major issues, but researchers became especially concerned that machines might never learn to parse textual meaning and select the right words in the first place. In a paper entitled “A Demonstration of the Nonfeasibility of Fully Automatic High Quality Translation,” Yehoshua Bar-Hillel declared that “no existing or imaginable program” (my emphasis) could ever enable a computer to resolve even basic semantic ambiguities that pose no problem to human speakers. He offered by way of example the following sentence:
Little John was looking for his toy box. Finally he found it. The box was in the pen. John was very happy.
According to Bar-Hillel, no amount of surrounding context would give a computer the ability to pick the correct meaning of “pen” (an enclosure where small children can play), since a computer is not “an intelligent reader” with the world knowledge common to humans from a young age.
By 1966, ALPAC—a scientific committee tasked with evaluating the prospects of “automatic language processing”—was unanimous and unforgiving in their assessment, and recommended a drastic reduction in funding. “Unedited machine output from scientific text is decipherable for the most part,” they acknowledged, “but it is sometimes misleading and sometimes wrong (as is postedited output to a lesser extent), and it makes slow and painful reading.”
Though the ALPAC report slowed down research in machine translation, the much greater affordability of hardware components in the 1980s helped jump-start new work. Rule-based systems such as the one pioneered at Georgetown were replaced by statistical phrase-based systems. Statistical machine translation works by compiling large vocabulary tables, based on available multilingual corpora, and using algorithms to establish and match patterns in the source text. The progress of this technology has been steady, and sufficient—at least in some languages and for some types of text—to make machine-translated text something many professionals feel they can work with.
Alongside machine-translation proper, the translation industry has been revolutionized by Computer-Aided Translation (CAT) tools, software products that enable translators to work collaboratively, automatically storing their work into bilingual tables. When a new sentence comes up for translation, a CAT system will try to find a partial or perfect match within its memory and offer the stored translation as a suggestion, for the human translator to accept or tweak. More than a mere productivity aide, CAT tools can make translators more prone to working in small sentence units, instead of looking at entire paragraphs and deciding how best to split them into the target language to produce a native-sounding text (sentences in English, for instance, tend to be shorter than in Italian or French). That this happens to suit the needs of machine translation providers looking to acquire neatly aligned multilingual corpora demonstrates how fuzzy the separation between machine and human translation can become.
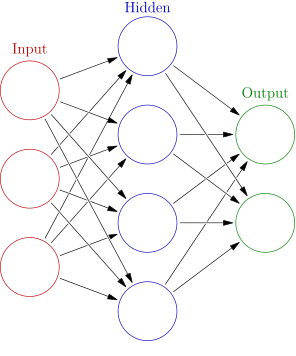
Neural networks and translation paradoxes
This was the state of the art until mid-2016. Machine translation was useful to many, but the progress of statistical systems appeared to have stalled. What we have witnessed since then is the shift to a new paradigm, as the world’s tech giants—Google, Facebook, Baidu, Amazon, Microsoft—lined up to adopt system based on self-learning neural networks. This is the story told by Gideon Lewis-Kraus and by Google’s marketing department, as the company was the first to declare victory in the war against human translators.
Using neural networks to perform machine translation is not a new idea, but it had new life breathed into by recent advances in the design and manufacture of graphics cards, which are used for machine learning systems for the same reason they are used to mine for bitcoin: they can make a lot of calculations very fast. A neural network is a computing system inspired by the prevailing model of human cognition: given an input, the nodes of a neural network, its “neurons,” work in unison, modulating their responses to produce the desired output. Image recognition is one of the most well-known current applications of these systems: by feeding the network a very large number of images already labelled by humans, the network will “learn,” over time, to recognize patterns. All the images that have been tagged as containing cats, for example, or all the photos have been tagged as containing your face; if the training is successful, when it is presented with a new image the network should be able to detect the underlying patterns and infer that it contains a cat. (Or your face.)
The network trained to make inferences about visual inputs can be trained to make inferences about textual inputs. A pattern is a pattern is a pattern, and it’s all numbers to a computer. A neural machine translation system, then, will be fed a large number of both monolingual and multilingual information, and will learn to recognize its patterns all on its own. It will then try to reproduce those patterns on command.
A good illustration of the fact that a neural network is engaged not merely in translation, but in a kind of textual production, comes from an experiment conducted by Andrej Karpathy using a recurrent neural network. Karpathy fed the network with the prose works of William Shakespeare, then asked it to produce an output, as opposed to translating an input, essentially instructing the network “now continue in this vein.”
The network complied:
Why, Salisbury must find his flesh and thought
That which I am not aps, not a man and in fire,
To show the reining of the raven and the wars
To grace my hand reproach within, and not a fair are hand,
That Caesar and my goodly father's world;
When I was heaven of presence and our fleets,
We spare with hours, but cut thy council I am great,
Murdered and by thy master's ready there
My power to give thee but so much as hell:
Some service in the noble bondman here,
Would show him to her wine.
This kind of text has a bizarre, nonsensical originality, and it goes on and on and on. Were all human life on Earth suddenly obliterated, you could imagine bots talking to themselves like this for as long as their power supply lasts, each hallucinating in their given specialist language, like so many parodies of speaking, thinking people. If it’s not parody, it’s imitation, which is not a bad word to deploy in a discussion about translation. Given a sufficient amount of training data produced by human translators, a recurring neural network is able to imitate what a human translator would do with a new source text and be reasonably effective at it.
Let us go back to the example given by Yehoshua Bar-Hillel to support his contention that computer could never master translation, Little John’s missing toy box. Google Translate currently renders it in Italian as follows:
Little John stava cercando la sua scatola dei giocattoli. Finalmente l’ha trovato. La scatola era nella penna. John era molto felice.
In French:
Little John cherchait sa boîte à jouets. Finalement, il l’a trouvé. La boîte était dans le stylo. John était très content.
In Spanish:
Little John estaba buscando su caja de juguetes. Finalmente lo encontró. La caja estaba en el corral. John estaba muy feliz.
And finally, in Chinese (simplified):
小约翰正在寻找他的玩具盒。 最后他找到了它。 盒子在笔中。 约翰很高兴。
Three of the four translate “pen” as “writing implement,” but Bar-Hillel was wrong; in Spanish, “corral” means both a literal livestock enclosure and a figurative enclosure where small children can play. The thing that could never happen has happened, if only once. And if it happened once, it means it can happen again, and happen more often.
The translations aren’t very good, of course. Translate was likely fooled by the capital “L” and interpreted “Little” as part of the boy’s name, like the character from Robin Hood. In all three romance languages, Translate forgot that the thing John was looking for was a box by the time it reached the second sentence, assuming instead that must be a generic item of masculine gender (whereas scatola, boîte and caja are all feminine). The Italian tenses are all over the place, indicating again that Translate has very poor retention of the linguistic choices it has made in the very recent past. (And yes, in three of the four languages Translate also thought that John found his toybox inside a writing implement.)
These mistakes render the text unusable; the amount of post-editing required would simply be greater than translating the passage from scratch, which is not far from the conclusions ALPAC reached in 1966. As computer scientists Yonatan Belinkov and Yonatan Bisk have put it, “synthetic and natural noise both break neural machine translation.” Neural networks can go too far in their search for patterns, finding language and meaning where there is none. This can happen as a result of so-called natural noise (when the input is corrupt) or be triggered by noisy information introduced on purpose, which also goes under the suggestive name of “adversarial training,” a practice designed to test the resiliency of AI models or simply mess with them.
You can try this at home. As Language Log contributor Mark Liberman discovered, Google Translate is liable to mistake random strings of vowels and spaces as Hawaiian, which it will dutifully attempt to translate. Indeed, I entered “aaa ii ooeou iiioe” and Translate returned “the solution to the problem.” In another post from the same series, Liberman muses, in a fashion reminiscent of Borges:
There were 361 distinct characters in my first input, so the number of different orders of different subsets of that input is very large. (…) Can we prove that any given English-language string is NOT the output for some permutation of one of those subsets?
The answer, I suspect, is that we can’t.
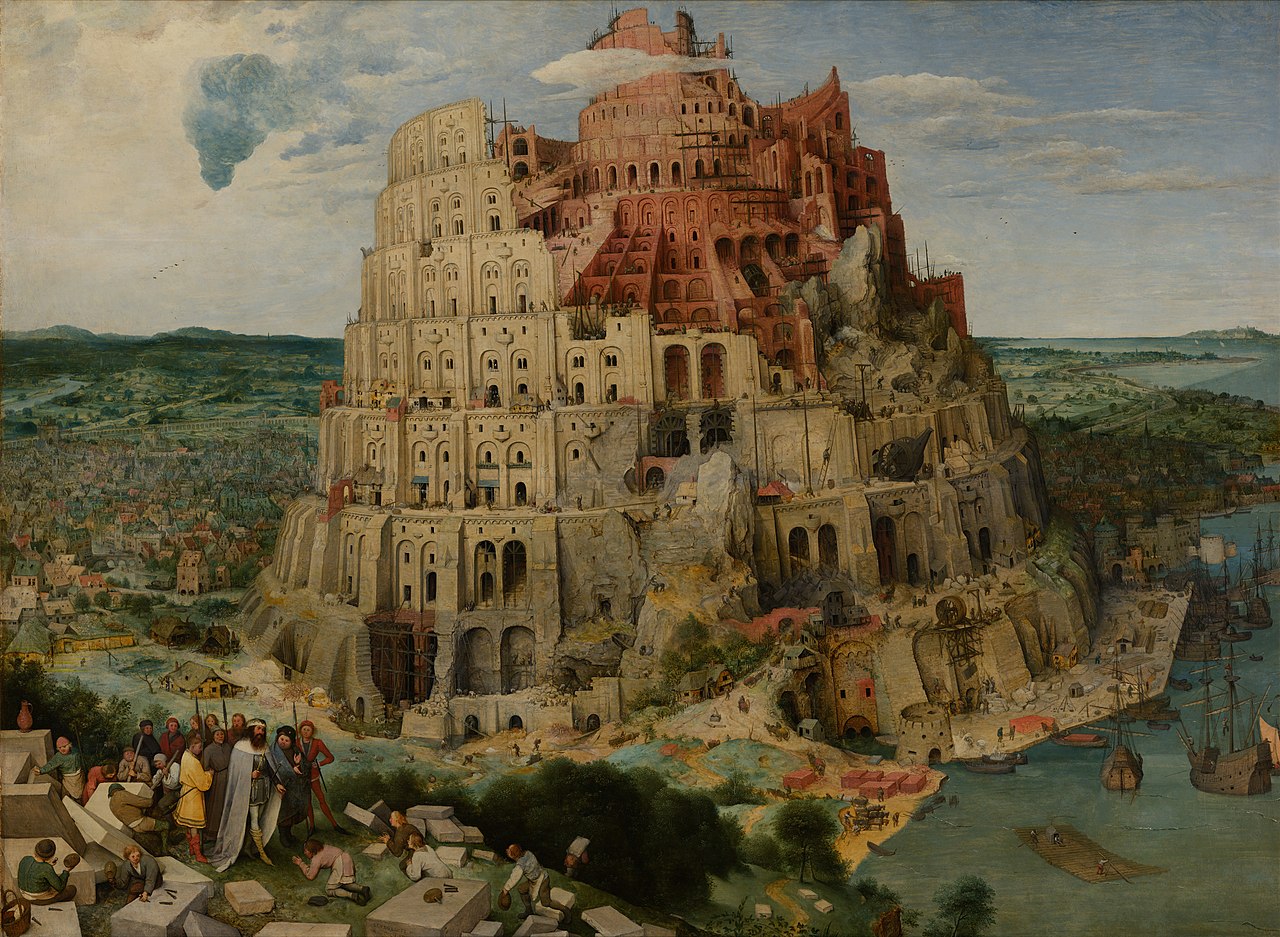
The fluent machine
We wouldn’t be having this conversation if machine translation didn’t do some things quite well, of course. For example, these are the first few lines of Jorge Luis Borges’ 1941 short story “The Library of Babel”; processed on February 1, 2018 by Google Translate:
The universe (which others call the Library) is composed of an indefinite and perhaps infinite number of hexagonal galleries, with vast air shafts between, surrounded by very low railings. From any of the hexagons one can see, interminably, the upper and lower floors. The distribution of the galleries is invariable. Twenty shelves, five long shelves per side, cover all the sides except two; their height, which is the distance from floor to ceiling, scarcely exceeds that of a normal bookcase. One of the free sides leads to a narrow hallway which opens onto another gallery, identical to the first and to all the rest.
This, by contrast, is the classic translation by Anthony Kerrigan used in the 1965 John Calder edition of Fictions.
The Universe (which others call the Library) is composed of an indefinite, perhaps infinite number of hexagonal galleries. In the center of each gallery is a ventilation shaft, bounded by a low railing. From any hexagon one can see the floors above and below—one after another, endlessly. The arrangement of the galleries is always the same: Twenty bookshelves, five to each side, line four of the hexagon’s six sides; the height of the bookshelves, floor to ceiling, is hardly greater than the height of a normal librarian. One of the hexagon’s free sides opens onto a narrow sort of vestibule, which in turn opens onto another gallery, identical to the first—identical in fact to all.
There are no two ways about it: the fluency of Google’s draft is remarkable. The text is plainer than Kerrigan’s, which may or may not be a good thing, depending on your preference. It contains only one mistake, but it’s a very interesting one: where Kerrigan correctly reported that “the height of the bookshelves, floor to ceiling, is hardly greater than the height of a normal librarian,” Translate writes “of a normal bookcase”—a puzzling discrepancy, given that the Spanish clearly says librarian (‘…de un bibliotecario normal’). My best guess is that Translate thought that this was a strange word choice, that it didn’t fit the pattern. In other words, it was expecting another word, decided that the author must be mistaken, and then acted accordingly.
This is something that has been observed: neural machine translation rates more highly for fluency than for accuracy. This is obviously problematic; editors can be called upon to correct a text for grammar and style, and to resolve semantic ambiguities, but what if the machine takes strange and unexpected liberties with the text?
Nevertheless, one has to be impressed with the improvements that machine translation shows in this example; ten years ago I saved a copy of the story translated by Babelfish and it was a mess. Consider the sentence above on which Google Translate stumbled: Babelfish got that detail right, but almost nothing else.
The distribution of the galleries is invariable. Twenty shelves, to five long shelves by side, cover all sides two less; its height, that is the one of the floors, exceeds as soon as the one a normal librarian.
The convergence of marketing messages, the system’s new-found fluency and the allure of AI quickly produced a powerful echo-chamber of hype, against which sceptical voices have been hard to make out. In late 2016, for example, when the new version of Google Translate was rolled out in Japan, it was greeted by a collective gasp as user after user stumbled upon its sudden, remarkable effectiveness. Google had neglected to make a formal announcement, supposedly to see how people would react—but also to garner the viral publicity effect. Within hours, Translate was the country’s top trending topic on Twitter.
Trying to temper the hype, Professor Andy Way of Dublin City University has noted that the universal translator is likely to be invented last of all the technologies that we remember as kids from Star Trek (including teleportation, phasers, and warp speed). But the loudest contrarian voice probably belongs to Douglas Hofstadter, who earlier this year ripped into Google Translate and its cheerleaders in a long article for The Atlantic. A cognitive scientist who has long supported the idea that computers could someday achieve human-like intelligence—as well as the author of Le Ton beau de Marot, a prolonged meditation on the art of translation—Hofstadter rolled out a long catalogue of Translate’s failures to adequately render a variety of texts as part of his own personal experiments. Computers are still not being taught to understand language, he argued, but merely to parrot it. More than that, Hofstadter is not only scathing of machine translation as a product, but horrified by machine translation as a project:
I am not in the least eager to see human translators replaced by inanimate machines. Indeed, the idea frightens and revolts me. (…) If, some “fine” day, human translators were to become relics of the past, my respect for the human mind would be profoundly shaken, and the shock would leave me reeling with terrible confusion and immense, permanent sadness.
In assigning to translation an ineffable, almost sublime quality (“To me, the word “translation” exudes a mysterious and evocative aura”), Hofstadter elevates all translators to the heights of Svetlana Geier. However, I can personally attest that many of us spend most of our time dealing with texts and topics that are much more mundane. I believe in the value of a good technical translation, but I don’t feel the need to appeal to the mysterious aura of my craft in order to advocate for it.
There is a more basic flaw in Hofstadter’s argument than the recourse to turgid rhetoric. He introduced his topic by referring to two highly educated acquaintances of his—one a Danish speaker who is fluent in English, the other an English speaker who understands Danish—who together have chosen, much to his puzzlement, to communicate with each other via Google Translate. Hofstadter never mentions this fact after the prologue, nor tries to explore their motivations. Yet the scenario offers an implicit, powerful counter-argument: people use machine translation tools even if they are imperfect, and often with full knowledge of their imperfections. They use them because they help them to communicate, or because they see value in expressing themselves in their own language, rather than attempting imperfect translations of their own.
“Appealing to a single notion of quality—human translation quality—no longer makes any sense,” as Andy Way said to me; “when one evaluates the quality of a machine translation system, you must ask yourself: quality for what?” It’s important to be suspicious of the hype, but what of those myriad daily decisions to resort to the services of a daft but immensely powerful machine?
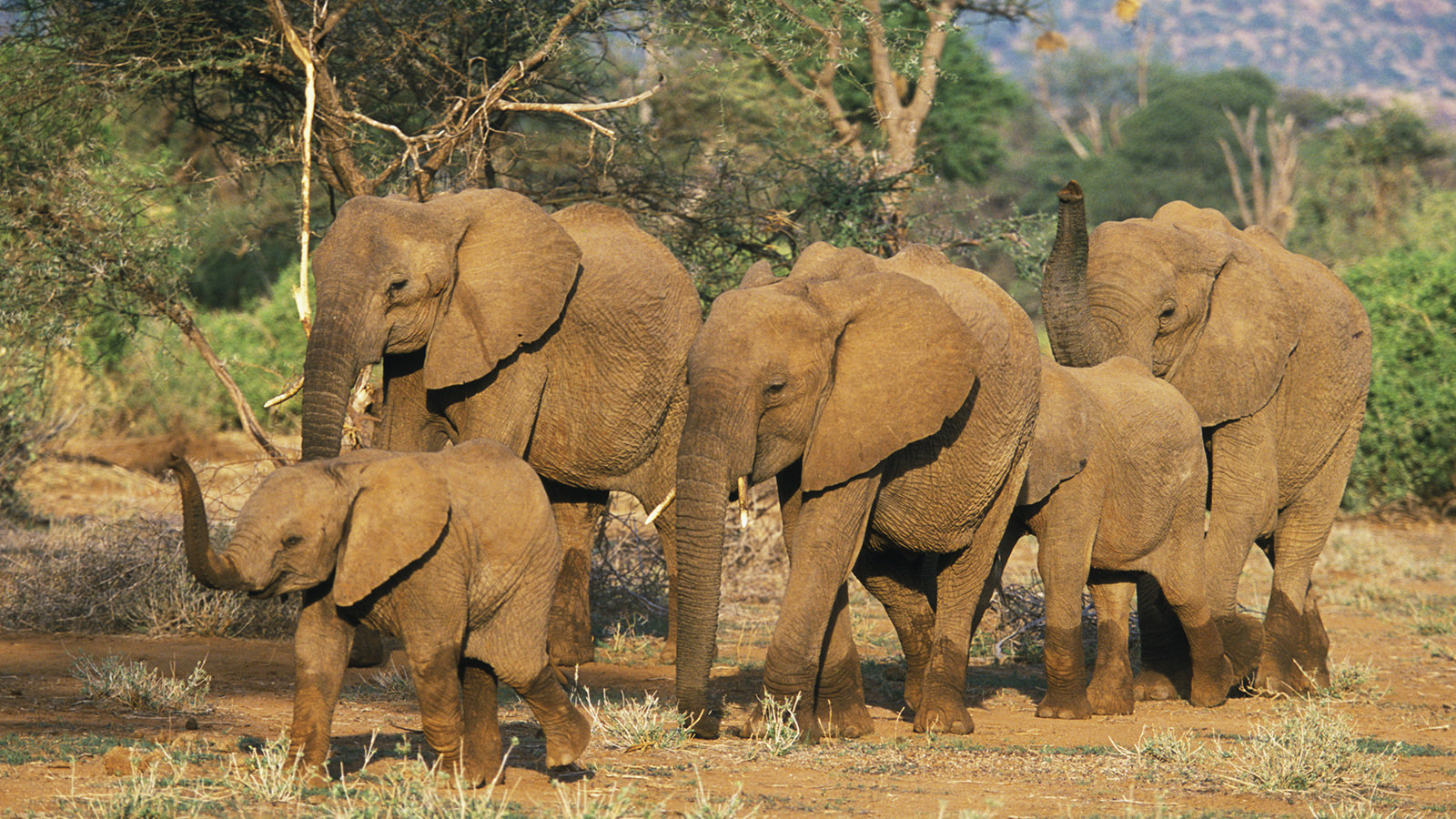
Conclusion: The future of the thing we call translation and the people we call translators
When it comes to the future of human translators, most commentators have settled for a narrative that people in my profession will find reassuring. Dr Marco Sonzogni, reader in translation studies at Victoria University of Wellington, put it very pithily when he described to me the trajectory between machine and human translation as “asymptotic.” Yes, machines will pre-translate more and more texts, and be better and better at it, but so too will the volume of translated texts increase, creating more work for translators. While some have claimed that technical translators may become redundant within ten years, there seems to be a consensus—however fragile and provisional—that the gap is not about to be bridged.
Personally, I am not so sure, and can easily envisage a near future in which the computer-aided tools of the trade carry out so much of the work themselves as to make the contributions of translators, if not altogether redundant, at least far harder to account for and remunerate. At the moment, most translators get paid by the word, and offer discounts to their clients on repeated text from previous work. A machine-translation engine trained on my own translations for a particular client could learn to take care of the bulk of a new text and, mimicking my own style, churn out all of the ‘easy’ sentences, the ones that I can translate as fast as I can type anyway. I would then be left to translate the ‘hard’ ones, which is where the value I add resides anyway, but for far less money than before. This could result in the bottom of the industry—which is global, non-unionised and almost entirely comprised of freelancers—to fall out in fairly short order.
As for the future of “translation” itself, it appears at this moment to be in the hand of players like Facebook and Google—both of which are primarily in the business of advertising, and have a staggering capacity to bend public behaviours and perceptions to their commercial needs. Think of the verb “to google,” impervious to translation: at most it will change shape, like in the Italian “googlare,” but always meaning nothing less than “to search for information quickly and efficiently.” Think of how words such as “friend” or “like” have been put to new, and arguably impoverished uses. Already there is a new word, gisting, which describes the act of obtaining the salient points of a text without having to resort to careful analysis. Why should words like translate and translation and translator be immune from similar shifts? How, when Google Translate alone processes over 140 billion words per day, and Facebook translates all foreign words put in front of its two billion active users as a default behaviour, unless instructed otherwise?
None of these developments are neutral, or immune from the power of the institutions that—as Lewis-Kraus has said—are being built around us, to say nothing of those that have been there the whole time. No single episode is more emblematic in this respect than the arrest by Israeli police in October of last year of a Palestinian worker after he wrote ‘good morning’ in Arabic above a photo of himself leaning against a bulldozer, and Facebook translated it into ‘attack them’. The man was detained and questioned for several hours before he managed to explain himself. At no point before his arrest—as the Guardian tersely reported—did any Arabic-speaking officer read the actual post. It wasn’t Facebook or machine translation that did this: the conditions for the ‘bad’ use of these tools were already in place.
The challenge, then, is to put machine translation to good use, whether it is to learn things about language and cognition from its interesting mistakes; to peer into previously marginalised literatures, in a way that might foster proper dissemination and study; or to devise systems—as it is being done—for international responders to operate more effectively in disasters and crises. All of these uses require a willingness to advocate for the full value of translation itself as a human activity, not only when it is practiced by an aged translator who carefully weighs every word of a literary masterpiece, as if she had all the time in the world: but in its most humble form, when it is driven by anyone with a desire to know or make public what is being said by people who speak another language.
When translation is viewed as a cultural project, there is no shortage of work that could be undertaken, nor of people needed to carry it to fruition.
***
With many thanks to Stephen Judd, for several conversations and for pointing me to the Language Log posts.